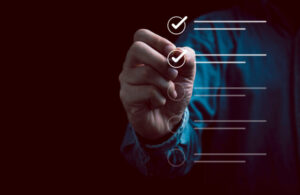
[Adobe Stock]
In the following interview, UpDyke explains how a holistic quality management strategy in pharma can help manufacturers adhere to the industry’s strict regulatory standards. Such an approach can help pharma companies avoid problems related to sub-standard ingredients, possible contamination, or a temperature excursion during delivery, all of which can jeopardize the integrity of the entire product.
In the following interview, UpDyke also explores the impact of digital transformation on the industry, and the benefits of integrating artificial intelligence (AI) and high-quality data to enhance quality assurance.
How can a holistic approach to quality management increase operational efficiency and profit margins?
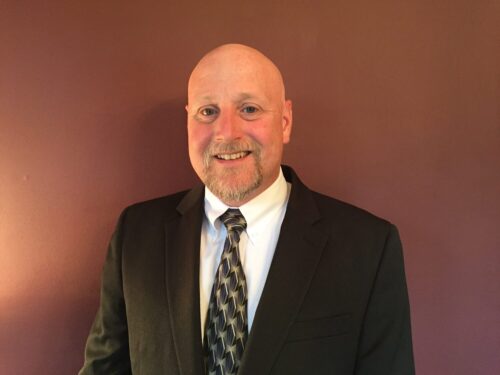
Dan UpDyke
UpDyke: We are currently in the era of Pharma 4.0, the ISPE initiative that aims to bring Industry 4.0-based manufacturing concepts to pharma. This evolution is characterized by digital transformation and the generation of enhanced data in terms of quality, variety, and availability across production systems. One of the key advantages of this transformation is the achievement of end-to-end visibility and precise control over the entire production and supply chain ecosystem. Adopting a holistic approach to quality management drives various factors that can enhance operating efficiencies and profit margins.
A proactive risk management strategy is an essential component of a holistic quality management approach. Proactively identifying and addressing risks throughout the product lifecycle can minimize downtime, mitigate potential losses, and enhance operational efficiencies, thereby supporting profit margins. Furthermore, a comprehensive and robust quality management system can optimize and streamline workflow processes, detect potential issues such as product defects and production bottlenecks, and ultimately improve the bottom line.
Pharma manufacturers can experience several benefits, including reduced defects, fewer product recalls, decreased waste, shorter production times, and faster time to market. Additionally, real-time monitoring of system performance, coupled with data analysis, enables manufacturers to identify areas for continuous improvement, driving incremental enhancements in operational efficiency and quality. Over time, this leads to increased profitability and reduced costs.
Digital transformation equips pharma manufacturers with a wide range of tools to operate with maximum efficiency and gather the necessary data to meet regulatory and audit requirements. These tools encompass equipment control software management, sample selection, certification of analysis, process monitoring data, and building management systems. Adhering to good manufacturing practices (GMP) and good distribution practices (GDP), as well as implementing a holistic quality management system, is crucial for ensuring regulatory compliance. By doing so, manufacturers can avoid penalties, product recalls, disruptions to operations, and potential damage to their reputation caused by non-compliance issues and product-related problems.
How can pharmaceutical manufacturers apply a holistic approach to areas such as research and development, supply chain, or distribution processes?
UpDyke: In R&D, pharma manufacturers can adopt Quality by Design (QbD), a concept that emphasizes designing quality into a product and recognizes that most quality issues stem from the initial product design. Applying QbD principles during the R&D phase entails mapping out critical quality attributes, process parameters, and conducting risk assessments from the early stages of development.
Comprehensive documentation, and even digitalization, of R&D activities, protocols, design, testing, and outcomes facilitates knowledge transfer, ensures traceability, and supports regulatory compliance. Furthermore, collaboration and communication among R&D, manufacturing, and quality assurance teams foster alignment on quality requirements, enabling proactive identification of potential quality issues.
When it comes to the supply chain, as mentioned earlier, establishing a robust supplier qualification program is crucial. This program involves assessing capabilities and quality systems, continuously monitoring and managing supplier performance to ensure adherence to quality standards, and supporting holistic quality management. Utilizing supply chain management and visibility tools enables the tracking and tracing of products throughout the distribution process. Effective supply chain management facilitates the identification of risks such as disruptions, ingredient availability issues, and non-compliant suppliers, allowing for the implementation of contingency plans.
Adhering to Good Distribution Practice (GDP) guidelines ensures the quality, safety, and integrity of pharma products during storage, handling, and distribution. It is essential to implement robust procedures, temperature controls, and monitoring systems to maintain product quality throughout the distribution network. Additionally, a robust process for handling adverse events, non-conformities, and product complaints should be in place. Thorough investigation, documentation, and appropriate reporting play a vital role in maintaining holistic quality management.
How does a comprehensive view of quality in IT infrastructure impact the overall quality assurance in pharmaceutical manufacturing?
UpDyke: Maintaining complete visibility of the IT infrastructure is essential for supporting regulatory compliance and ensuring that systems are designed and implemented to meet regulatory requirements. This includes areas such as data integrity, electronic record handling, rights access, traceability, and regulatory reporting. A comprehensive view of quality also ensures the correct implementation and maintenance of electronic documentation management systems, such as electronic batch records (eBR), electronic document management systems (EDMS), and electronic quality management systems (eQMS).
Process automation and control is an area where full visibility of the IT ecosystem significantly impacts quality assurance, as these systems monitor and regulate manufacturing operations. Manufacturing execution systems (MES) and process control systems incorporate validation, change control, and system checks to ensure that automated processes maintain the necessary quality standards. Integration of data and capture of operator activities enables electronic batch records and reduces manual paper-based operations, reducing error and simplifying compliance.
The IT infrastructure plays a crucial role in managing and storing critical data associated with product formulations, manufacturing processes, quality control tests, and regulatory compliance in pharmaceutical manufacturing. A comprehensive perspective on quality in the IT infrastructure supports robust data integrity and security measures, safeguards data accuracy, prevents unauthorized access, and ensures compliance with data integrity regulations.
Can you provide examples of the tools available to pharma manufacturers today that can enable quality management beyond the production floor?
UpDyke: Pharma manufacturers employ several tools beyond the production floor to support quality management. Enterprise Resource Planning (ERP) systems integrate various business functions, including quality management, providing real-time visibility into manufacturing, supply chain, inventory, and quality data. These systems streamline processes, manage supplier quality, track material movements, and enable end-to-end traceability across the entire value chain.
For managing laboratory processes and data, there is Laboratory Information Management Systems (LIMS) software designed specifically for this purpose. LIMS solutions enable sample tracking, instrument integration, test method management, result recording, and data analysis. They enhance data integrity, reduce manual errors, and ensure compliance with good laboratory practices (GLP) and good manufacturing practices (GMP).
Electronic Quality Management Systems (eQMS) provide centralized platforms for managing and automating quality processes across an enterprise. They enable electronic document control, deviation and corrective and preventive action management, change control, training management, and audit management. eQMS systems streamline quality-related activities, enhance traceability, and facilitate compliance with regulatory requirements.
Track and trace technologies, such as serialization and barcode systems, play a vital role in monitoring and tracing pharmaceutical products throughout the supply chain. These technologies help prevent counterfeiting, ensure product authenticity, and enable efficient recall management.
How does the integration of higher quality data and AI technologies contribute to holistic quality assurance in pharmaceutical manufacturing?
UpDyke: In pharma development and manufacturing, integrating higher quality data and AI technologies leads to numerous advancements.
By integrating and analyzing higher quality data collected from various sources throughout the manufacturing process, AI can identify patterns, trends, and correlations on a large scale that may not be easily discernible to humans. This capability provides valuable insights for informed decision making. For instance, AI can identify root causes of quality issues, optimize process parameters, and recommend and implement preventive measures.
AI algorithms continuously analyze data from system devices, enabling the identification of any deviations and triggering alerts or automated adjustments to maintain quality standards. This minimizes the risk of product non-conformance. AI also optimizes manufacturing processes to enhance quality control. Machine learning (ML) algorithms analyze data to identify optimal process parameters, critical quality attributes, and relationships between different elements. This leads to the development of advanced process control strategies like model predictive control (MPC) systems, allowing real-time adjustments and optimization of process variables for improved quality outcomes.
Predictive analytics is another critical area where AI provides operational intelligence. By comparing real-time data against historical patterns, process parameters, and environmental conditions, AI, supported by ML algorithms, identifies deviations that may cause quality issues and predicts potential future quality outcomes. AI models various quality issue scenarios and recommends preventive actions, proactively avoiding quality deviations, reducing rejections, and improving overall product quality.
The integration of AI and high-quality data also supports continuous improvement by facilitating the capture, analysis, and sharing of knowledge. AI algorithms mine data for insights, identify areas of improvement, and provide recommendations for process enhancements. This knowledge becomes the foundation for ongoing process optimization, training programs, and quality management strategies.
Tell Us What You Think!