
[max_776/Adobe Stock]
In the pharmaceutical industry, operational issues from efficiency losses to failed batches can delay delivery of potentially lifesaving therapies to patients. For this and other reasons, pharmaceutical organizations require resources dedicated to batch prediction, planning and rapid issue resolution throughout the entirety of a drug’s lifecycle. Because the field is both highly regulated and consequential, decisions must be data-based to ensure compliance and product integrity.
Adding to the challenge, patient populations are ever-evolving and conditions in the supply chain are variable. At the same time, there is growing pressure to lower the cost of bringing drugs to market, without compromising safety and efficacy. Responding to these trials requires embracing digitalization in manufacturing and regulatory settings.
Advanced analytics can help pharmaceutical processors adapt to the changing needs throughout drug development, empowering engineers to streamline common workflow challenges faced by the pharmaceutical industry, and ultimately save time and money. This article will explore some of the challenges advanced analytics technologies help automate and overcome, supported by two success stories.
Overall equipment effectiveness
Pharmaceutical companies have a wide variety of key performance indicators (KPIs) at their fingertips to quantify and track equipment efficiency. One popular metric for quantifying downtime and equipment limitations is overall equipment effectiveness (OEE). This calculation accounts for otherwise unidentified system constraints—such as excess equipment shutdowns—by factoring in availability, performance and quality.
It is not sufficient to simply run monitoring and planning systems in the background because results and impacts must be visible among all levels of an organization. For OEE and other data to provide value, it must inform actions on the shop floor.
Removing siloes among operations, engineering and management staff can accelerate results, allowing key stakeholders to collaborate more efficiently. Using OEE, these key process personnel can quickly gain insight into remediation recommendations for efficiency losses, fostering rapid root cause analysis and corrections in near-real time.
The need for OEE
There is often a discrepancy between engineer-executed OEE calculations and actual conditions on the manufacturing floor, such as equipment micro-stoppages and delayed communication of downtime events. However, understanding these conditions and their contributors in near-real time is essential for accurate assessments and root cause analyses, which help prevent future failures.
When pharmaceutical manufacturing organizations experience equipment or line limitations but lack strategic, systematic ways to interpret effectiveness metrics, OEE data can help pinpoint causes. Many standard approaches for OEE implementation require significant manual time investments parsing out and organizing downtime data, associating reason codes and attributing operational context to specific events.
Advanced analytics solutions provide insights
Advanced analytics solutions address these manual processes by automatically assigning reason codes to downtime issues, categorizing efficiency losses for further analysis and standardizing visualizations. Additionally, multilevel reason codes provide drill down analysis for rapid root cause identification, with manual event categorization and timely subject matter expert (SME) commentary when needed.
This flexibility empowers operational and engineering personnel to collaboratively identify disruptive circumstances that may not get caught in post-batch data review. While downtime data provides useful information to help SMEs increase throughput and optimize efficiency, this information can also be fed into batch records to provide critical input on unexpected batch interruptions.
Combinations of graphical, tabular and chart visualizations allow for ease of information sharing among plant personnel, and summary statistics—like downtime duration—can be broken down by reason code and shared in interactive dashboard formats. This makes it easy for stakeholders to dive into more technical details of each analysis as needed, with access to data and summary reports tailored to specific stakeholder levels and needs.
Advanced analytics technologies can be configured to notify SMEs when downtime events occur, visualized in both dashboard and trending views, which has a breadth of implications. One example is informing organizations of unexpected downtime periods experienced at a contract manufacturing organization (CMO) facility during a high stakes production campaign (Figure 1).

Figure 1: A downtime event at a contract manufacturing organization, displayed in both dashboard and trend view.
Figure 1: A downtime event at a contract manufacturing organization, displayed in both dashboard and trend view.
Leveraging OEE to reduce downtime
A global, top-10 pharmaceutical company leveraged OEE analysis using the strategy described previously to monitor and improve their lines, resulting in a $65M per year increase in production revenue. With easy drill-down analysis and time savings from faster root cause identification, SMEs have since redirected their time to higher-level efforts, and they also benefit from better understanding of the processes with insight into micro-stoppages that would otherwise be ignored. A variety of visualization tools provide staff at all levels with transparency into the process and access to the data required to make informed operational decisions.
For organizations spending time and money on manual OEE tracking and trending, automation can provide million-dollar savings opportunities, unlocked by incremental improvements in process efficiency and OEE data. This capital can be directed toward more productive tasks, such as new drug discovery initiatives, manufacturing facility expansions and additional SME resources.
Critical quality attributes and the golden batches
In addition to OEE data, pharmaceutical companies also frequently depend on critical quality attribute (CQA) testing for batch release. Lab methods of long duration and testing with slow results often cause late identification of failed batches post-production.
Take, for instance, an encapsulation process relying on post-production acceptable quality limit (AQL) testing for release. While routine in-process checks attempt to catch and correct glaring deviations from target parameters and ranges, some issues cannot be resolved or captured on the production floor. Without easy access to a slew of historical data and near-real time comparisons of historical profiles, plant personnel are often blindsided by failed batches identified post-production.
For this reason, organizations must integrate downstream lab data with real-time processing data to generate predictive insights. Using golden batch tools with advanced analytics solutions, SMEs are empowered to generate and analyze batches in near-real time against historical trends, and proactively mitigate and correct bad batch occurrences.
Using golden batches to identify bad batches in near-real time
Pharmaceutical organizations are increasingly leveraging golden batch tools for this reason, but these instruments often require significant personnel resources for model maintenance. Hours can be spent sifting through historical batch data for each critical process parameter (CPP) to generate an adequate reference profile for future use.
Armed with advanced analytics solutions, however, SMEs can perform a one-time selection of golden batches—batches that remained within specifications on CQAs and within action limits on CPPs. These batches can then be embedded into a model to generate reference profiles against which past and real-time batches can be compared.
Using the standard deviation, mean and other statistics of these datasets, SMEs can proactively identify excursions in near-real time to catch and correct bad batches before it is too late. Process data can then be normalized and overlaid for easy comparison between batches and against the golden batch limits.
Leveraging CQA and CPP analysis
A top-30 American biotechnology company saved more than 10 hours per parameter per lot in production analyses by deploying golden batch modeling. The company previously relied solely on diacetyl level lab results to differentiate good from bad batches, but test result availability often lagged batch production by several days. Diacetyl level was retroactively plotted alongside the associated CPP, temperature, and if diacetyl fell beneath a certain threshold, the batch was released.
By using the analytical capabilities in the Seeq, the organization began correlating batch properties with historical diacetyl test results to proactively differentiate good from bad batches. Continuous automated analysis also provided the ability to ascribe golden averages, minimums and maximums to all batches.
In the solution, SMEs can review quality data and select good batches on which to generate reference profiles. With batch data overlaid and normalized to start at the same time, SMEs can easily identify bad batches and apply limits to these while in progress, correcting them without needing to wait for diacetyl testing results.
Figure 2: Process trend and lab data from individual batches (top), overlaid to produce a reference profile represented by the dotted line (bottom).
Speeding up safe drug delivery
With mounting pressure to manufacture drugs more rapidly, efficiently and cost-effectively, new digitalization solutions must be embraced to achieve these tall orders. Fortunately, advanced analytics solutions enable rapid insights from time series process data, and by integrating lab and manufacturing data in a single location, SMEs can save time that would otherwise be spent compiling and sifting through vast quantities of information.
With technologies tailormade for parsing through complex manufacturing floor reason codes, organizations can track and trend OEE metrics, with manual intervention on an as-needed basis. And instead of waiting days or even weeks, on batch quality lab data, SMEs can utilize golden metric profile tools to monitor CPPs in near-real time.
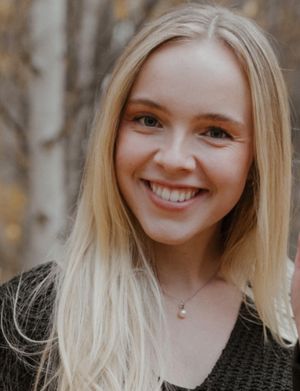
Tatum O’Kennedy
These advances are empowering organizations to make batch-saving decisions on the plant floor, reducing waste and increasing development speed. Pharmaceutical organizations across the board — including CMOs, non-CMOs, R&D, commercial, development and manufacturing science and technology organizations—are leaning on digital resources to monitor process data throughout the drug life cycle, enabling better insights for quality metrics and delivering lifesaving drugs to patients more quickly.
All figures courtesy of Seeq.
About the Author
Tatum O’Kennedy is an analytics engineer at Seeq. After graduating from Northeastern University with a master’s in chemical engineering, Tatum worked in a variety of roles in the pharmaceutical industry, including formulation development of small molecules, product development of spray-dried dispersions and multiparticulates, and manufacturing science and technology engineering for drugs undergoing commercialization. In her role at Seeq, Tatum continues to combine her passion for the pharmaceutical industry with a love for analytics, and works with pharmaceutical companies to generate the most value from their time series data.
Tell Us What You Think!